Between July and August 2024, the ATHENA Christian Doppler Laboratory hosted four interns working on the following topics:
- Halime Lezi: Image and Video Compression Pipeline
- Luka Kaiser: VidStream
- Julius van Dillen: Enhancing Video Quality with Super-Resolution
At the conclusion of their internships, they presented their work and results, receiving official certificates from the university. This collaboration was mutually beneficial for both the researchers at ATHENA and the interns. Their learning process was enhanced by the dedicated guidance they received, which included personalized mentorship, hands-on training, and continuous support. This comprehensive supervision ensured that they not only developed practical skills but also gained a deeper understanding of the research methodologies and technologies used in the video streaming field. We extend our gratitude to all three interns for their genuine interest, productive efforts, and valuable feedback on the laboratory.
Halime Lezi: “I had an awesome time during my four-week internship at ATHENA. My project was about image and video compression, and I learned a lot about how it works. I also got to use Python, which was both fun and challenging. The work environment at ATHENA was really supportive and interesting. My supervisor, Emanuele Artioli, was super helpful and always ready to answer my questions. He made sure I understood both the practical and theoretical parts of my work, which was really cool. It was also great to work with people from different countries. The team was friendly, and we got along well. The work-life balance was good, with a nice mix of work and relaxation. Overall, my time at ATHENA was very educational and enjoyable. The skills and knowledge I gained during this internship will be really useful for my future studies and career. I’m thankful for the opportunity and the support I received. I would highly recommend this internship to anyone looking for a rewarding and fulfilling experience. It’s a great place to learn, grow, and meet new people.”
Luka Kaiser: “I had an amazing four weeks at ATHENA. It was really nice meeting new colleagues and even making new friends. The project I worked on was exactly what interests me, and if I had any questions, my supervisor, Christian, was always there to help me out. So, thank you very much for that. Overall, this experience has been incredibly valuable, and I learned a lot and gained practical skills that I will definitely use in the future. My time here was both productive and enjoyable. I am grateful for the opportunity and would love to stay connected with everyone I’ve met. Thank you once again for everything.”
Julius van Dillen: “My four-week internship at ATHENA was incredible. I focused on improving image and video quality through Super-Resolution techniques. I had the opportunity to work with a variety of tools and technologies, including FFmpeg, Visual Studio Code, Python, several Super-Resolution architectures, and various video quality metrics. I was truly surprised by how much Super-Resolution enhances video and image quality. I really enjoyed working with these technologies and found the entire process fascinating. I am grateful to have had Daniele as my supervisor; his guidance and support made the experience both easier and more enjoyable. During my internship, I gained valuable insights into the research process and the fundamentals of Python programming.”
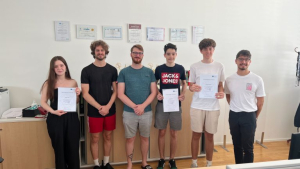